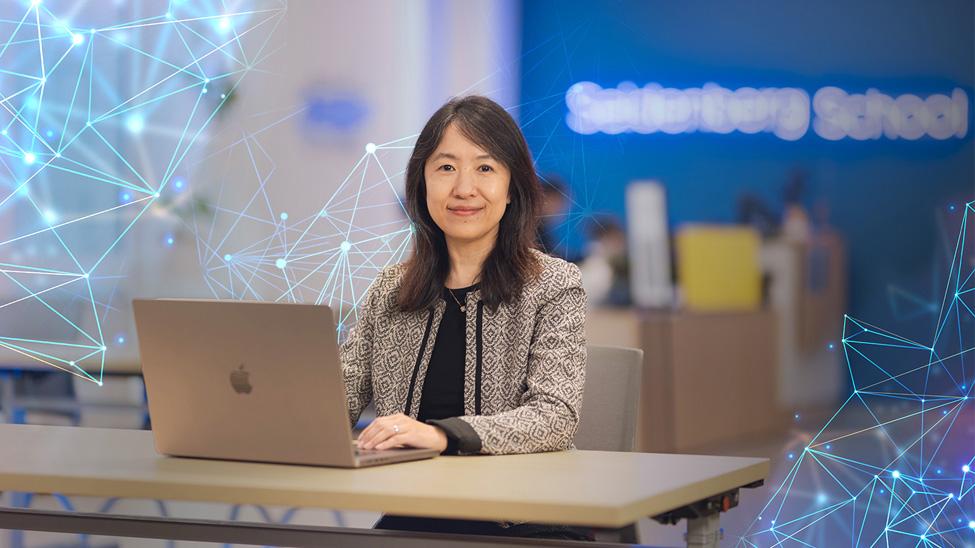
Smart Medicine: The Promise and Peril of AI in Healthcare
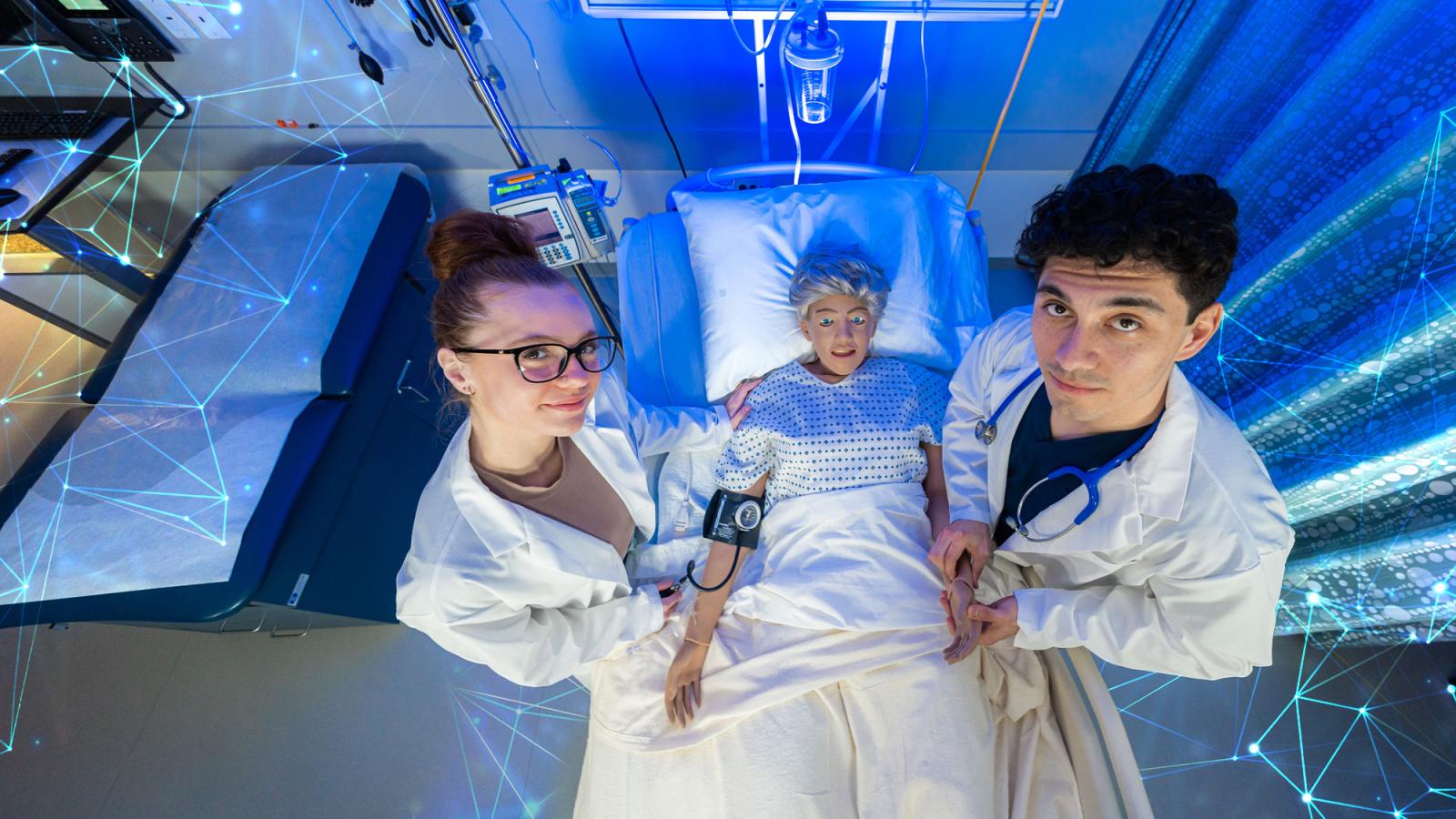
To the untrained eye, the grainy medical images vaguely look like knees, black and white scans of what might be muscle, bone, and green wisps of something else.
But to Juan Shan, PhD, an associate professor of computer science in the Seidenberg School of Computer Science and Information Systems at Pace University, the photos are validation of a decades-long hunch: robots can read an MRI.
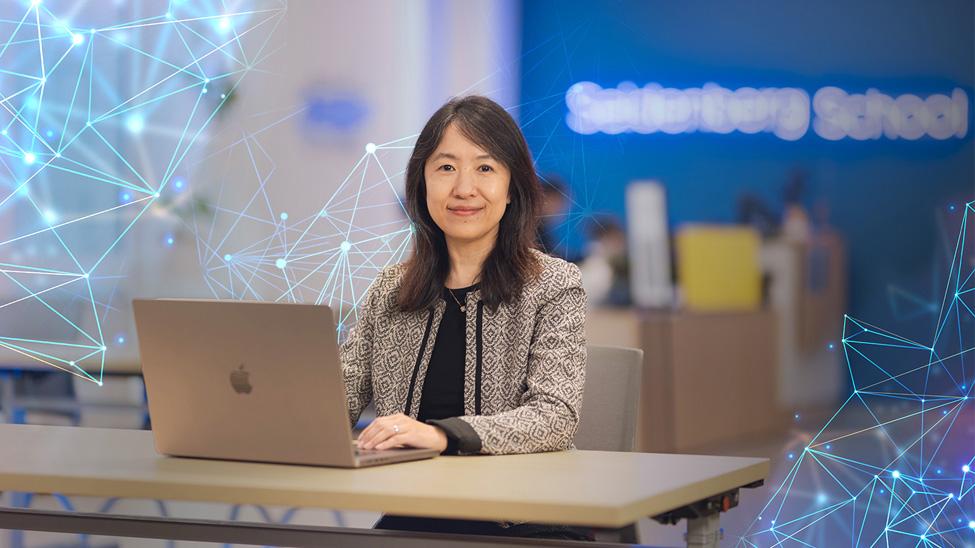
“The method does not require any human intervention,” Shan wrote in a recent paper detailing her machine learning tool for identifying bone marrow lesions (BMLs), early indicators of knee osteoarthritis. In a standard MRI, BMLs appear as pixelated clouds. In Shan’s model, they pop in vibrant hues of color.
“This work provides a possible convenient tool to assess BML volumes efficiently in larger MRI data sets to facilitate the assessment of knee osteoarthritis progression,” Shan wrote.
As artificial intelligence (AI) reshapes how medicine is practiced and delivered, Pace researchers like Shan are shaping the technology—and the guardrails—driving the revolution in clinical care. Computer scientists at Pace harness machine learning to build tools to reduce medical errors in pediatric care and strengthen clinical decision-making. Social scientists work to ensure fairness and transparency in AI-supported applications. And students are taking their skills to the field, addressing challenges like diagnosing autism.
Collectively, their goal isn’t to replace people in lab coats. Rather, it’s to facilitate doctors’ work and make medicine more precise, efficient, and equitable.
“In healthcare, AI enables earlier disease detection, personalized medicine, improves patient and clinical outcomes, and reduces the burden on healthcare systems,” said Soheyla Amirian, PhD, an assistant professor of computer science at Seidenberg who, like Shan, trains computers to diagnose illnesses.
“New York is a world-class hub for innovation, healthcare, and advanced technologies, and its diversity makes it the perfect place to explore how fair and responsible AI can address inequities across populations,” Amirian said.
In Shan’s lab, that work begins below the kneecap. Together with colleagues, she feeds medical images—MRIs and X-rays—into machine learning models to train them to detect early signs of joint disease. They’re looking to identify biomarkers—cartilage, bone marrow lesions, effusions—that might indicate whether a patient has or is prone to developing osteoarthritis, the fourth leading cause of disability in the world. Current results indicate her models generate results that are highly correlated with manual labels marked by physicians.
“We want to apply the most advanced techniques in machine learning to the medical domain, to give doctors, radiologists, and other practitioners a second opinion to improve their diagnosis accuracy."
Shan’s vision is to create diagnostic tools that would supplement human interventions and pre-screen patients who are at lower risk of disease.
“We want to apply the most advanced techniques in machine learning to the medical domain, to give doctors, radiologists, and other practitioners a second opinion to improve their diagnosis accuracy,” she said. “Our goal is to automate time-consuming medical tasks—like manual labeling of scans—to free doctors for other, more human tasks.”
Pace has invested heavily in training future leaders in AI and machine learning applications. A key focal point for these efforts has been in the healthcare sector, where rapid innovations are changing the patient experience for the better. Over the last decade, Pace researchers have published more than 100 papers in peer-reviewed journals addressing questions in psychology, biology, and medicine. Much of this work has taken advantage of AI applications.
Information technology professor Yegin Genc, PhD, and PhD student Xing Chen explored the use of AI in clinical psychology. Computer science professor D. Paul Benjamin, PhD, and PhD student Gunjan Asrani used machine learning to analyze features of patients’ speech to assess diagnostic criteria for cluttering, a fluency disorder.
Lu Shi, PhD, an associate professor of health sciences at the College of Health Professions, even uses AI to brainstorm complex healthcare questions for his students—like whether public health insurance should cover the cost of birth companions (doulas) for undocumented migrant women.
“In the past, that kind of population-wide analysis could be an entire dissertation project for a PhD student, who would have spent up to two years reaching a conclusion,” Shi said. “With consumer-grade generative AI, answering a question like that might take a couple of days.”
Pace’s efforts complement rapid developments in healthcare technology around the world. Today, AI is helping emergency dispatchers in Denmark assess callers’ risk of cardiac arrest, accelerating drug discoveries in the US, and revolutionizing how neurologists in Britain read brain scans.
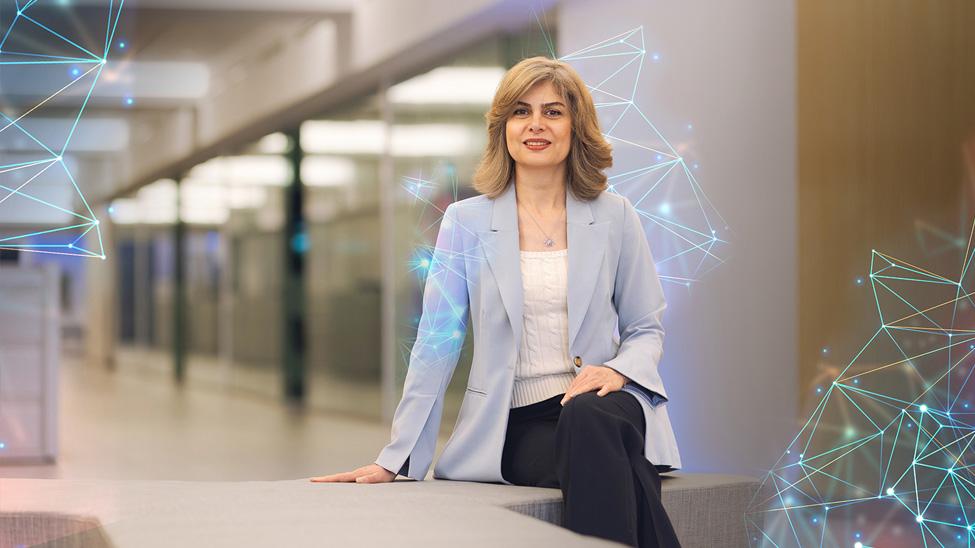
Amirian, like Shan, is developing AI-powered tools for analyzing the knee. Her work, which she said has significant potential for commercialization, aims to assist clinicians in diagnosing and monitoring osteoarthritis with accurate and actionable insights. “Its scalability and ability to integrate with existing healthcare systems make it a promising innovation for widespread adoption,” she said.
A key focus for Amirian is building equity into the algorithms she creates. “Reducing healthcare disparities is central to my work,” she said. As head of the Applied Machine Intelligence Initiatives and Education (AMIIE) Laboratory at Pace, Amirian leads a multidisciplinary team of computer scientists, informaticians, physicians, AI experts, and students to create AI models that work well for diverse populations.
Intentionality is essential. “The objective is to develop algorithms that minimize bias related to sex, ethnicity, or socioeconomic status, ensuring equitable healthcare outcomes,” Amirian said. “This work is guided by the principle that AI should benefit everyone, not just a privileged few.”
Zhan Zhang, PhD, another Pace computer science researcher, has won accolades for his contribution to the field of AI and medicine. Like Amirian and Shan, he shares the view that while AI holds great potential, it must be developed with caution. In a recent literature review, he warned that “bias, whether in data or algorithms, is a cardinal ethical concern” in medicine.
“Data bias arises when data used to train the AI models are not representative of the entire patient population,” Zhang wrote in a co-authored editorial for the journal, Frontiers in Computer Science. “This can lead to erroneous conclusions, misdiagnoses, and inappropriate treatment recommendations, disproportionately affecting underrepresented populations.”
“While AI offers immense opportunities, addressing challenges like algorithmic bias, data privacy, and transparency is crucial.”
Preventing bias in AI healthcare applications won’t be easy. For one, privacy concerns can create a bottleneck for securing data for research. There’s also a simple numbers challenge. Unlike AI models trained on public image benchmarks, which draw on millions of inputs, training AI models on medical images is limited by a dearth of information, said Shan. While there are efforts to augment the dataset and generate synthetic data, the relatively small size of the available medical datasets is still a barrier to fully unlocking the potential of deep learning models.
Solving these challenges will be essential for AI’s potential in healthcare to be realized. “While AI offers immense opportunities, addressing challenges like algorithmic bias, data privacy, and transparency is crucial,” Amirian said.
Simply put, AI is both a threat and an opportunity. “The opportunity lies in its potential to revolutionize industries, improve efficiency, and solve global challenges,” Amirian said. “But it becomes a threat if not used ethically and responsibly. By fostering ethical frameworks and interdisciplinary collaboration, we can ensure AI serves as a tool for good, promoting equity and trust.”
Above all, she said, as AI offers “smarter solutions” to many modern problems, it’s also “challenging us to consider its societal and ethical implications.”
More from Pace
AI is changing the world—but should we be worried? To test its ability to engage in real academic discourse, Pace's writers tasked ChatGPT’s Deep Research with generating a fully AI-written, cited academic article. By pushing its capabilities, we’re not just showcasing what AI can do—we’re interrogating its limitations.
From helping immigrants start businesses, to breaking down barriers with AI-generated art, Pace professors are using technology to build stronger, more equitable communities.
Pace University Professor of Art Will Pappenheimer, who has long incorporated digital media and new technologies into his artwork, discusses his latest AI-influenced exhibition and the technology’s effects on the art world.